In the world of market research, synthetic data is being positioned as a game-changer. AI-generated respondents promise faster and cheaper insights while eliminating traditional survey challenges like response bias, straight-lining, and panel quality issues. The idea that a machine can replicate consumer decision-making is compelling, but does it actually work? Can synthetic data truly predict consumer behavior with accuracy? And should brands trust AI-generated insights over real consumer feedback?
In our latest Between Two Joels session (watch the full recording here), our AI experts (aka. Chief Data Science Officer, Joel Anderson, and our VP of AI, Joel Armstrong), explored the potential and limitations of synthetic data.
What is synthetic data?
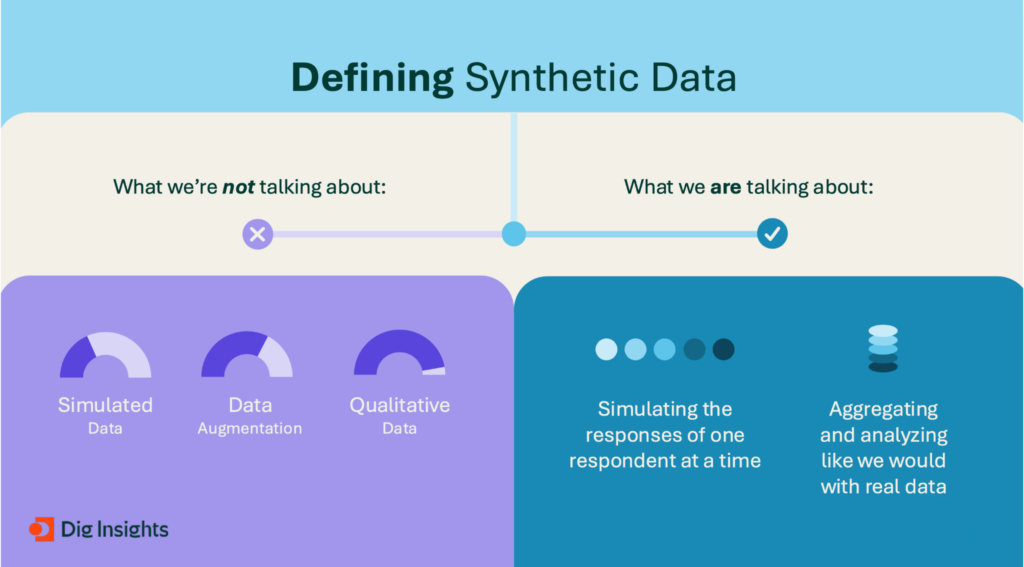
Synthetic data refers to AI-generated datasets that mimic real-world responses. Instead of collecting survey answers from actual consumers, large language models (LLMs) generate simulated respondents based on predefined characteristics such as demographics and preferences. While this may sound like an innovative way to streamline research, it’s important to clarify what synthetic data is and what it is not.
Unlike simulated data, which is often used to test models, or data augmentation, which fills in missing respondent segments, synthetic data aims to replace traditional survey respondents altogether. It functions by creating individual responses at scale, which can then be aggregated and analyzed just like real consumer data. On the surface, this offers the potential for significant cost savings and efficiency gains, but whether it delivers the same level of accuracy as human respondents is another question entirely.
The big promise vs. reality
Many synthetic data providers claim their AI-generated insights can replace traditional survey respondents, offering consumer insights that are faster, cheaper, and potentially (extra emphasis on potentially) even more accurate. If this were true, it would transform the industry by removing the need for expensive and time-consuming fieldwork. However, as Carl Sagan famously said, “Extraordinary claims require extraordinary evidence.”
To determine whether synthetic data lives up to its promises, the Joels designed a study that put it to the test. The goal was to see whether synthetic respondents could accurately predict box office performance for a selection of movies—a real-world scenario where consumer preferences directly translate into measurable success.
The study: can synthetic data predict box office performance?
We tested synthetic data to understand it’s capabilities and limitations by selecting 30 movies across three different years—2018, 2019, and 2023. The first two years were chosen because AI models likely had training data on them, allowing us to assess whether synthetic data could “backcast” past results. The year 2023 was selected as a blind test, ensuring that none of the movies’ final box office performances were included in the AI model’s training data.
Next, we generated 500 synthetic respondents using an LLM to create personas that matched real-world moviegoer demographics. These synthetic respondents were then asked to participate in our Upsiide idea screening method, where they swiped left or right on movie concepts to indicate interest and preference. Finally, we compared the synthetic respondents’ ratings with the actual box office revenue of the films to see if synthetic data could accurately predict success.
The results: where synthetic data succeeded (and failed)
The results revealed a stark contrast between backcasting (predicting past results) and forecasting (predicting future success).
When tested on movies from 2018 and 2019, AI-generated responses showed a strong correlation (0.85) with past box office performance. This suggested that synthetic data could effectively model historical consumer sentiment, as the AI had access to reviews, discussions, and general awareness of these films.
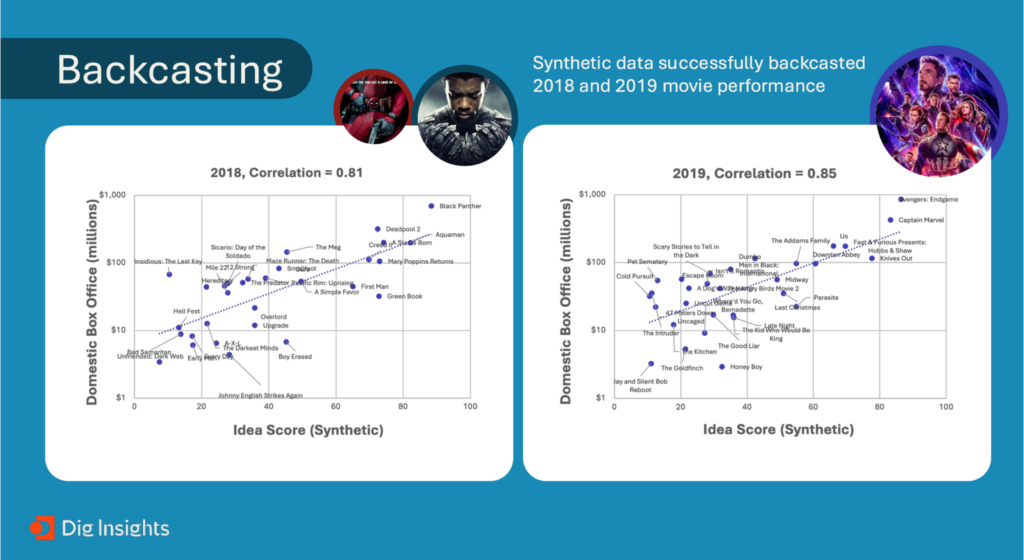
However, when applied to movies from 2023—titles that were not in the AI model’s training data—the results were much weaker. The correlation between synthetic respondents’ interest and actual box office revenue dropped significantly to 0.5. When we excluded sequels and remakes (which the AI might have indirect knowledge of from previous franchise entries), the correlation fell even further to just 0.3, barely above random chance.
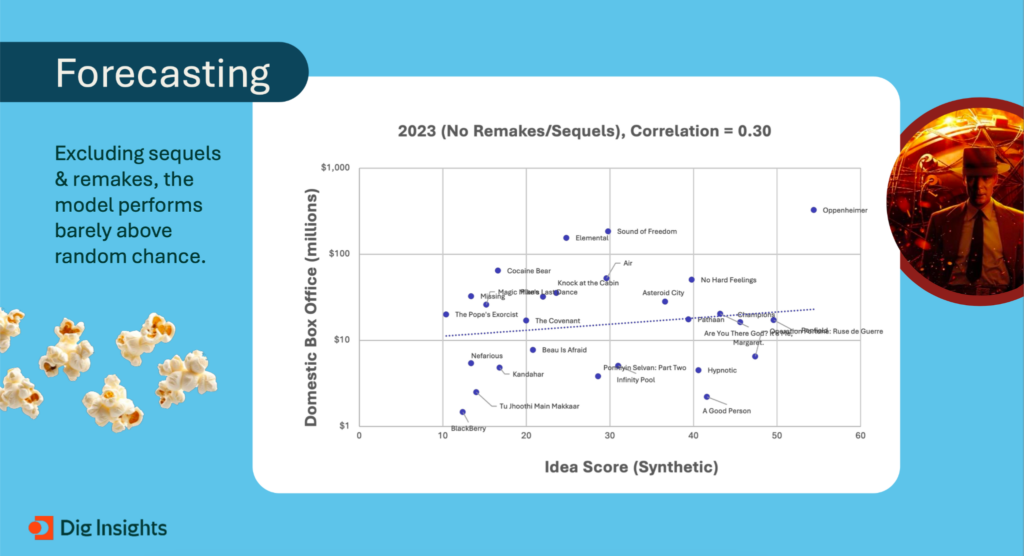
This finding highlights a fundamental limitation: synthetic data struggles to predict future success for new ideas, products, or movies that aren’t already widely discussed online. The AI simply lacked the necessary training data to generate accurate consumer responses for concepts it hadn’t “seen” before.
Limitations of synthetic data
Beyond its struggles with forecasting, synthetic data carries additional risks. One major concern is bias in subgroup representation. AI models learn from the internet, which means they inherit the biases of online discourse. As a result, synthetic data tends to overrepresent dominant groups while underrepresenting minority communities. If a company relies solely on synthetic respondents, they risk making business decisions based on skewed or incomplete insights.
Another issue is the lack of validation standards. There is no widely accepted framework for evaluating the accuracy of synthetic data, and many providers fail to offer transparency about their methodologies. Claims of “95% accuracy” sound impressive but often lack peer-reviewed evidence. Without rigorous validation, it is difficult for brands to trust AI-generated insights with high-stakes business decisions.
So, when (if ever) should synthetic data be used?
Despite these challenges, synthetic data is not without potential. While it is not yet ready to replace human respondents, there are certain scenarios where it may be useful. For example, it can be beneficial in early-stage idea screening, where researchers are looking for directional guidance before launching full-scale testing. It may also provide value in consumer perception studies, where the goal is to analyze well-established brands or products.
However, synthetic data should not be used for forecasting new product success, validating market demand for untested innovations, or making high-impact business decisions without real consumer input. At best, it should be seen as a complement to traditional research, not a replacement for human insights.
The future of synthetic data in market research
As AI technology evolves, synthetic data may become more reliable. Advances in fine-tuning models, reducing bias, and improving domain-specific training data could increase its accuracy. However, for now, real consumer feedback remains irreplaceable, particularly for businesses seeking to understand emerging trends and validate new ideas.

At Dig Insights, we are excited about the possibilities AI brings to market research, but we remain committed to human-led insights enhanced by technology. AI is a powerful tool, but it is just that—a tool. The best research outcomes come from leveraging AI to support, rather than replace, real consumer engagement.
What’s Next?
If you’re curious to learn more about how AI and synthetic data are shaping market research, check out our full Between Two Joels session, where we dive even deeper into the results of our study.