In October 2024 we brought together 50 of the best client-side researchers to talk all things AI. The topics of the day ranged from the use cases and trustworthiness of LLMs right through to how folks have implemented AI solutions effectively; with lots of knowledge shared by our in-house AI experts, the Joels.
Some of the key discussions that took place:
- While the appetite to use AI is there, the ‘how’ is still somewhat unknown.
- To successfully use AI you need to understand where the data is coming from and assess your options as Switzerland might.
- Insights leaders have a real opportunity to own AI as a part of their overall remit, but they’ve got to be proactive about it.
We shared our immediate takeaways from Buzz a few weeks ago but we wanted to dive even deeper on some of the more complex topics.
Understanding the AI Hype Cycle
At Buzz, we kicked off the day with an introduction to the AI Hype Cycle from our co-founder Michael Edwards.
The Hype Cycle is a methodology that delineates between hype and commercial viability and aims to represent the maturity, adoption, and social application of new technologies. It plots expectations over time, and maps the journey from innovation triggers, through inflated expectations, to the trough of disillusionment, to the slope of enlightenment and finally the plateau of productivity.
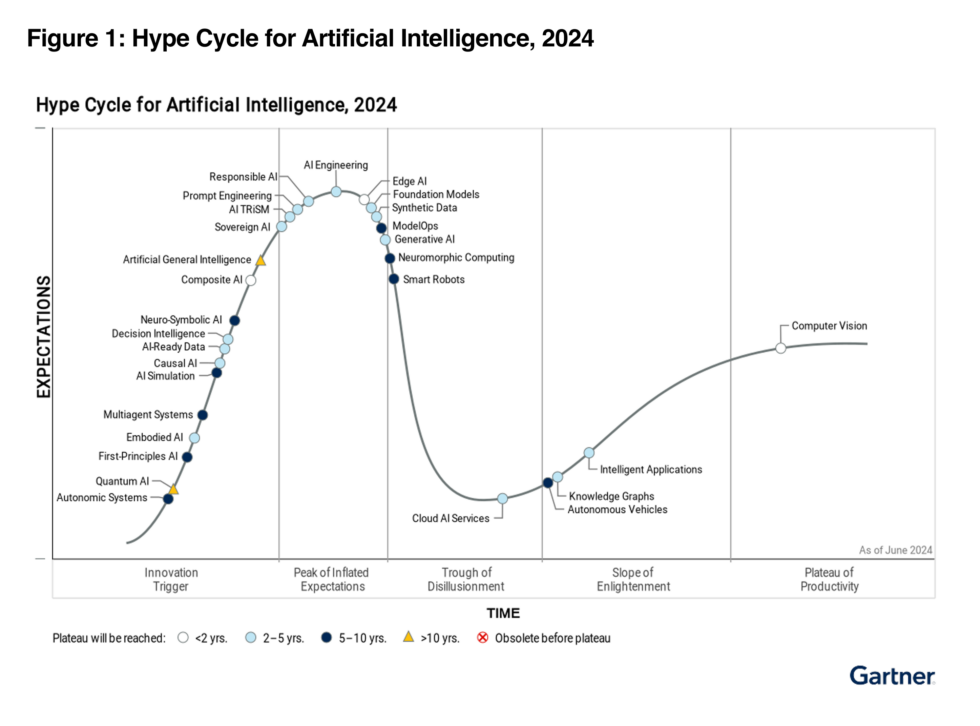
It’s important to understand where different AI applications land on the Hype Cycle before we begin talking about ways to adopt and implement them. Some of the more familiar AI applications like Generative AI and Prompt Engineering currently sit on or near the “Peak of Inflated Expectations”, with very few use cases represented past the “Trough of Disillusionment”.
In fact, only Computer Vision, a computer’s ability to identify and understand objects and people in images and videos, has made it to the plateau of productivity. Remember how fun it was to laugh at Facebook’s automatic face detection and tagging system for your photo dumps back in 2010?
Not every application shown above is relevant to the insights industry. We mapped Dig’s work and experiments with AI onto the Hype Cycle to better understand what researchers might want to invest time and resources in today, and where their focus for the future should be.
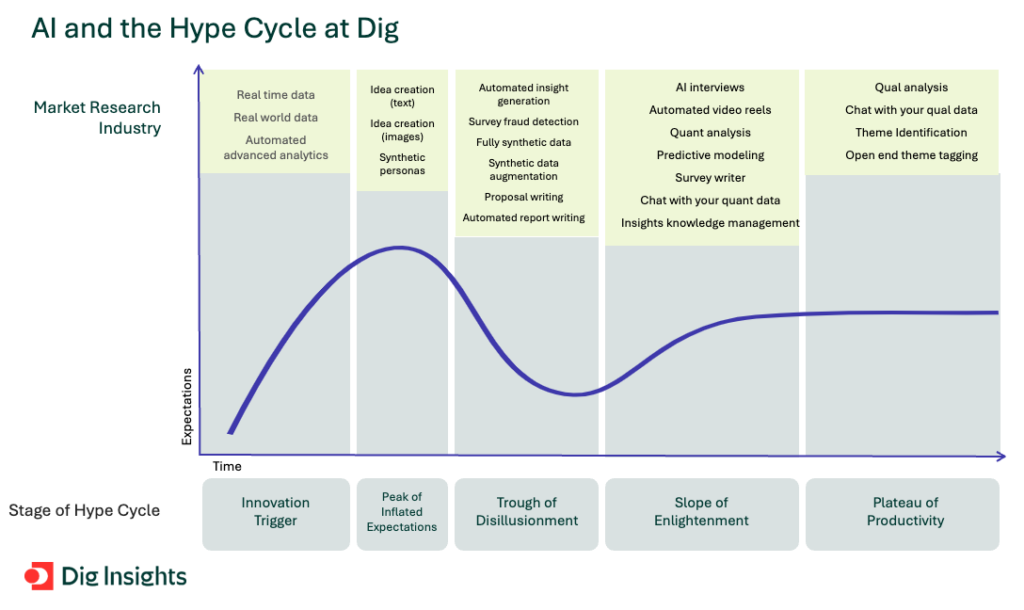
Some of the most used (and most useful) applications for AI at Dig are our analytical tools. This includes meta-analysis for themes, qual and quant analysis, and predictive modeling. While much of the complex meta-analysis is handled by the brilliant minds within our Advanced Analytics team, our proprietary in-house Open Ended Text Analytics Platform (OTAP) democratizes rapid AI analysis to our entire organization. It’s a walled garden that allows our researchers to “chat” with their data, including unstructured qualitative data, in an intuitive way.
We ran an experiment with OTAP to see if it could pull advertising themes out of verbatim responses. We found that OTAP correctly identified several themes, emotions, and feelings by analyzing memories shared by the American public; and that those themes mirrored the most popular sports advertising.
The elephant in the room: can I trust AI findings?
It’s important to approach the “tech topic of the moment” with caution, but AI isn’t going anywhere. Conversations at Buzz focused on how to bring rigor to vetting AI applications, empowering researchers to ensure the trustworthiness of AI usage.
We discussed implementing a series of checks and guardrails to ensure that any data you are generating with AI is trustworthy. Attendees agreed that they shouldn’t use AI on anything they can’t double-check or verify. We also discussed what it would look like to implement risk levels per product or industry type. Pharma? High risk – do not use AI. Salty cheese snacks? Lower risk – go ahead and start experimenting.
The insights teams have a responsibility to ensure the quality of the data they are providing, both in terms of innovation success and consumer trust. And that starts with your suppliers.
At Dig, we’re proud of not only our commitment to innovation but also our commitment to useful innovation. We take extra care to ensure any new algorithms, AI updates, or methodologies we share with our clients are built or validated by our Advanced Analytics team.
One topic we discussed at length at Buzz was synthetic data and its usefulness as an input to or replacement for survey data. Over the summer our team ran a series of experiments with synthetic data and shared the results publicly. We wanted to see if AI could go beyond modeling existing data and if it could really understand consumers to predict the unexpected, like the Barbenheimer craze of 2023.
Spoiler alert: we found that synthetic data could be useful for backwards-looking data (aka the years that have already happened) but struggles to predict future behavior. Read the full Whitepaper in our resources section.
Supplementing your current work with AI
Expectations for AI implementation are high, and you might even have a mandate to use AI in various forms in your daily work life. But if the cool, hot AI applications sit at the peak of inflated expectations (synthetic data and idea generation, for example) how can you build in elements of trustworthy, proven, and reliable AI to your work?
Our AI experts, Joel and Joel, are very clear about this point. You can get better at the things that are available to you like prompt engineering, data analysis, and generative AI.
Prompt engineering is the art (or science, depending on who you ask) of prompting your LLM chatbot (like Dig’s OTAP or ChatGPT) to give you the best answers possible based on your question.
The Two Joels (our AI experts) host regular Q&A sessions for anyone in the industry who is keen to level up their AI game. The topic of prompt engineering comes up in almost every session. A few key takeaways to whet your appetite:
- The importance of Prompt Libraries and Frameworks: Developing and using prompt libraries can significantly improve efficiency and consistency in AI interactions. Frameworks like the Co-star prompt help you to provide the right information to AI models, which lead to more accurate and useful outputs.
- Applications in Data Analysis: AI tools are being extensively used to analyze large volumes of unstructured data, such as open-ended responses from focus groups and surveys. These tools can efficiently summarize and filter data based on different themes and subgroups. But the analysis of quantitative data with multiple filters remains a challenge, and there are ongoing efforts to improve AI capabilities in handling the data sets.
- Addressing Prompt Limitations and Rejections: The two Joels discuss strategies for dealing with situations where AI tools refuse to perform tasks that they are technically capable of. It’s important to tweak prompts and provide specific roles or contexts to guide the AI effectively. For example: if you’re looking for places to see in a new city, specifying a role such as “travel guide” can yield more precise and relevant recommendations from the AI.
When it comes to data analysis the Dig team are already using OTAP (Dig’s proprietary Open Text Analytics Platform) in client work to deliver faster and more impactful data analysis – it’s particularly powerful when handling qualitative interviews.
For example, our work with La-Z-Boy used OTAP to identify themes from transcripts of the group discussions. The AI-powered thematic analysis helped to eliminate researcher bias, which is a critical factor when seeking to understand social norms and lifestyles.
We actually experimented with AI-generated credit card rewards, and put them to the test against human-generated rewards and asked consumers which were the most compelling. We bet you can’t guess which is which. You can check out the study on the public Upsiide dashboard.
And if you really want to know, find out which ideas were AI generated here.
The future of AI
Researchers have a lot of concerns about AI; can I trust the AI data? Will AI take my job? How do I use AI ethically? At Buzz, we learned that referring back to the Hype Cycle was a useful way to frame up AI advancements in their current state and understand what they might look like in the future.
AI is here to stay, in the way that computer programs, online surveys, and Microsoft Excel are here to stay. When a technology is this widely applicable and powerful it becomes embedded in workflows, and becomes second nature. In the not-so-distant future, you might regularly forget it wasn’t always readily available to you.
But for the here and now we need to learn how to use AI to supplement our current work while cautiously vetting ways for AI to replace existing insights processes and practices altogether. Many Buzz attendees agreed that elevating the influence of research within an organization is going to come down to insights professionals embracing AI in the right places, vetting each use case and approach accordingly. Given the skillset of insights leaders, their ability to ask the hard questions, and the fact that they are often skeptical by design, we’re well placed to push AI usage across the organization in the right direction.
You’re not alone on your AI journey – we’re here to help. Lean on your research partners to learn best practices but also look to the future to understand where we’re headed.